How AI is Revolutionizing Scientific Discovery
Remember the days when scientific discovery meant countless hours in the lab, painstakingly testing hypotheses and analyzing data? Well, those days aren't exactly over, but they're certainly changing. Artificial intelligence (AI) is reshaping the scientific landscape, turning what was once a slow, methodical process into a high-speed journey of discovery.
How is AI Supercharging Hypothesis Generation?
Imagine having a brainstorming partner with access to all the world's scientific knowledge, capable of making connections that might take humans years to discover. That's essentially what AI brings to the table when it comes to generating hypotheses.
AI systems, particularly those using machine learning algorithms, can analyze vast amounts of existing data to identify patterns and correlations that might not be immediately obvious to human researchers. This capability is particularly useful in fields like drug discovery, where AI can analyze biological data and suggest potential drug candidates that human researchers might have overlooked.
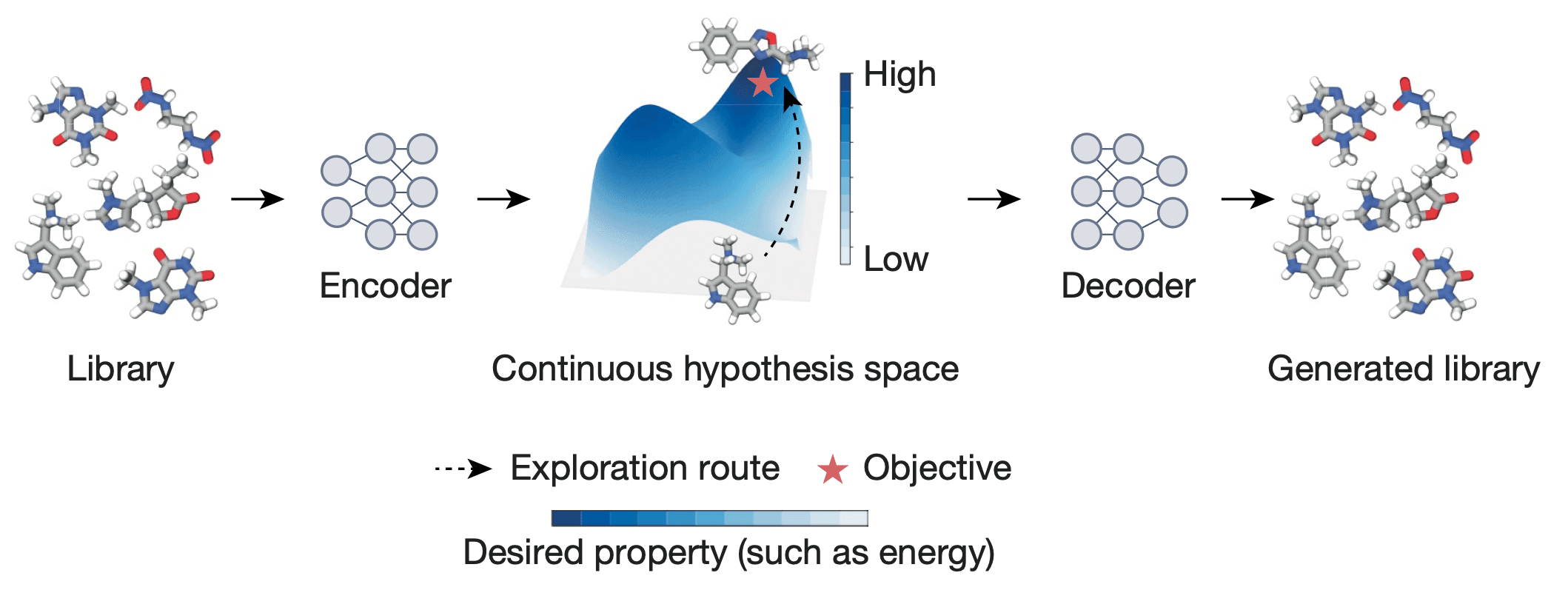
For example, as shown in the diagram above, AI can encode a library of chemical structures into a continuous hypothesis space. By exploring this space, AI systems can identify molecules with desired properties—such as energy levels or binding affinities—that meet specific experimental objectives. This approach allows researchers to navigate a broader range of possibilities more quickly and accurately [1].
But AI isn't just about crunching numbers. It's bringing a fresh perspective to the creative process of hypothesis generation. By combining knowledge from different scientific fields, AI can generate cross-disciplinary hypotheses that human researchers might not have considered. This interdisciplinary approach is crucial as scientific problems become increasingly complex and interconnected.
Can AI Really Design and Verify Experiments?
You bet it can! AI is not just coming up with ideas; it's also revolutionizing how we test them. Traditional experimental design often involves a lot of educated guesswork and time-consuming trial and error. AI changes this by using predictive modeling and automated optimization to streamline the process.
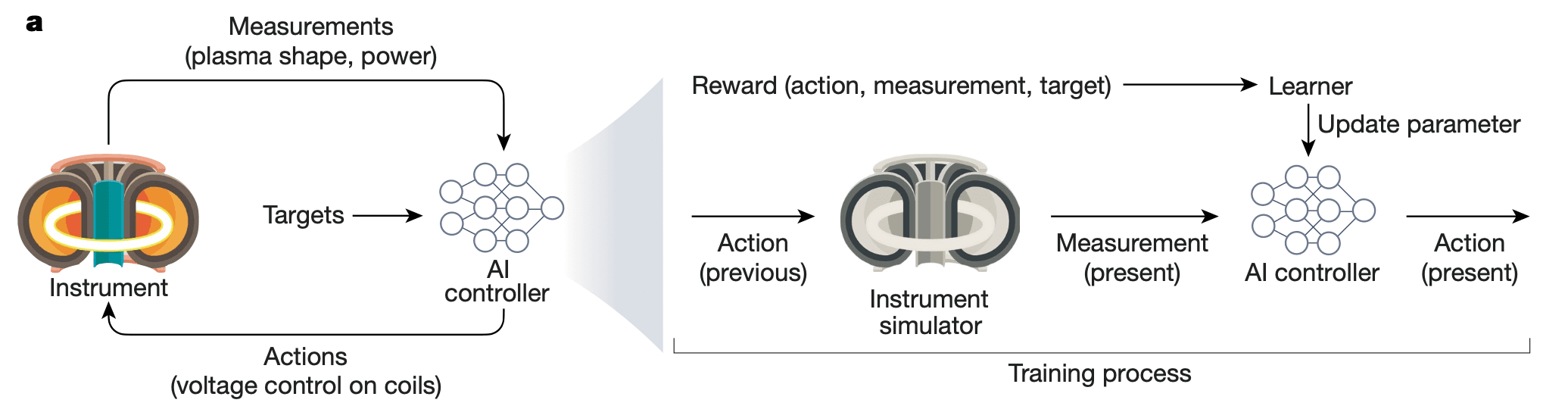
For instance, AI can control nuclear fusion experiments in a tokamak reactor. The AI controller receives real-time measurements of plasma shape and power, then adjusts voltage on coils to regulate magnetic fields and achieve the desired experimental conditions. Through simulations, AI learns to optimize its actions based on feedback, continually improving the reactor's stability and performance over time [1].
Instead of spending months tweaking different variables manually, AI uses machine learning algorithms to identify the parameters that will most likely impact the outcomes. This means scientists can focus on testing the most promising configurations rather than getting lost in endless iterations.
But AI's role doesn't stop at design. It's also a game-changer when it comes to verifying experimental results. AI-driven data analysis allows scientists to sift through large datasets quickly, identifying patterns, trends, and anomalies that might otherwise go unnoticed. Whether it's gene expression data or particle collision results, AI tools can provide insights that help scientists draw more meaningful conclusions.
What Are Self-Driving Labs, and How Are They Changing Research?
Picture a lab that never sleeps, tirelessly conducting experiments around the clock without human intervention. That's the essence of a self-driving lab. These autonomous research facilities combine AI, robotics, and advanced data analysis to design, execute, and analyze experiments independently.
Self-driving labs are particularly making waves in fields like chemical and materials sciences. They can explore a vast range of conditions and variables quickly, streamlining the experimental process in ways that were previously unimaginable [3].
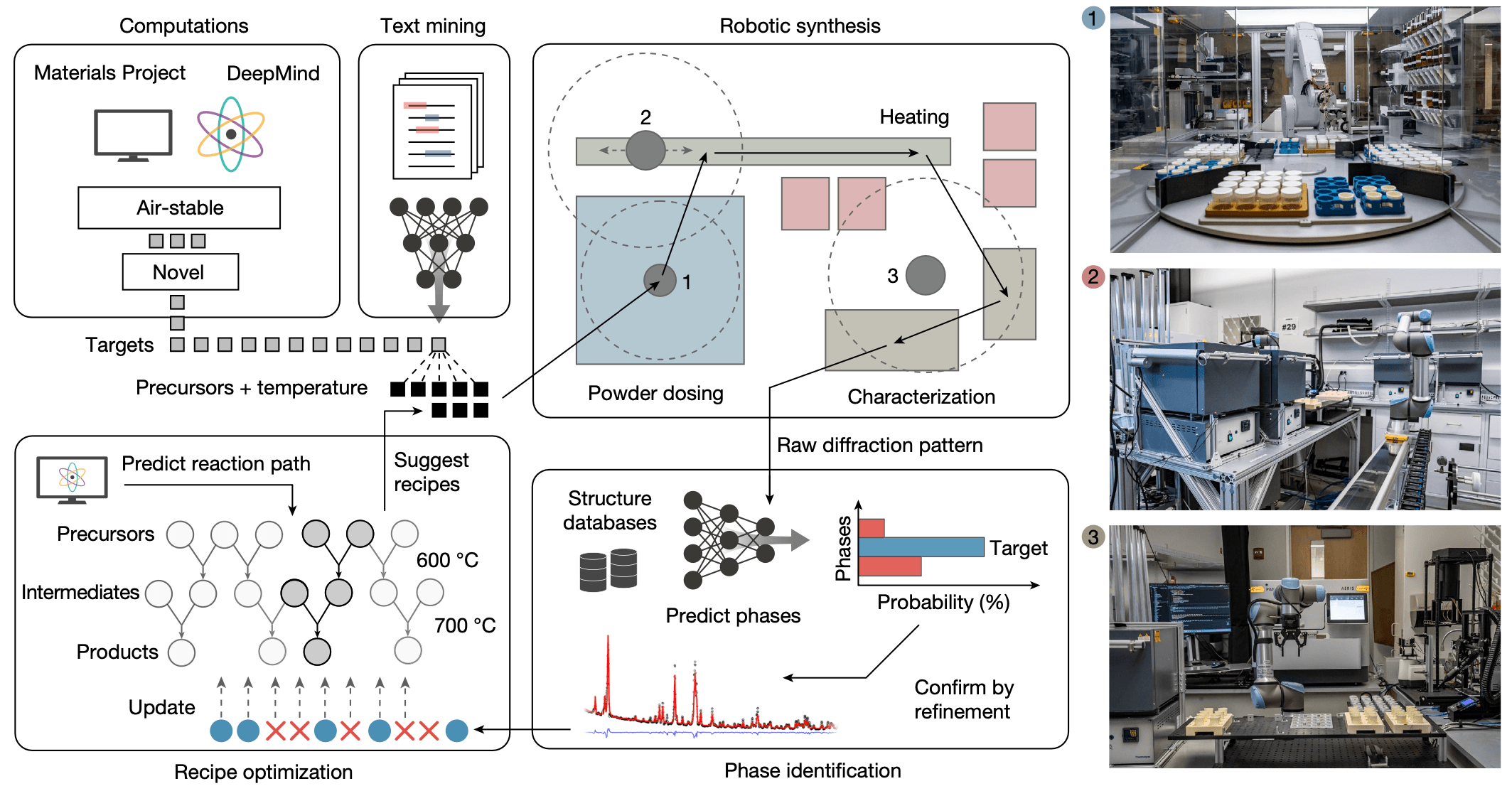
Scientists from the University of California, Berkeley and collaborators developed the A-Lab, an autonomous laboratory system for materials discovery and synthesis. This innovative platform integrates machine learning algorithms, robotic automation, and advanced data analysis to streamline the entire materials research process. The A-Lab identifies promising air-stable compounds, generates tailored synthesis recipes, and performs comprehensive characterization of new materials. By creating a closed-loop system from initial chemical input to final product analysis, the A-Lab accelerates the discovery and development of novel inorganic materials with potential applications across various technological domains [2].
What Challenges Does AI Face in Scientific Research?
While AI offers tremendous benefits, it's not without its challenges. One of the main hurdles is ensuring the quality and representativeness of the data used to train AI models. If the data is biased or incomplete, the AI might generate misleading results or overlook important factors.
Another challenge is the "black box" nature of some AI systems, particularly deep learning models. In fields like medicine, where understanding the reasoning behind results is critical, this lack of transparency can be a significant barrier.
Integrating AI into existing scientific workflows also presents challenges. Laboratories may need to invest in new technology and training to make the most of AI's capabilities. There's also the question of how to balance AI-driven research with traditional scientific methods and human intuition.
What Does the Future Hold for AI in Scientific Discovery?
As we look to the future, the role of AI in scientific discovery is only set to grow. We can expect to see even more sophisticated self-driving labs, capable of tackling increasingly complex research questions across a broader range of scientific disciplines.
AI is likely to become an indispensable tool for addressing some of our most pressing global challenges, from developing new sustainable materials to finding cures for diseases. As AI systems become more advanced, they may even start generating novel scientific theories, pushing the boundaries of human knowledge in unexpected ways.
However, the future of AI in science isn't about replacing human researchers. Instead, it's about creating a powerful synergy between human creativity and machine efficiency. By handling routine tasks and processing vast amounts of data, AI frees up scientists to focus on big-picture thinking, interpretation, and asking the kind of innovative questions that drive science forward.
References
- Wang, H., et al. (2023). Scientific discovery in the age of artificial intelligence. Nature, 620(7972), 47-60.Link
- Szymanski, N. J., et al. (2023). An autonomous laboratory for the accelerated synthesis of novel materials. Nature, 624(7990), 86-91.Link
- Abolhasani, M., & Kumacheva, E. (2023). The rise of self-driving labs in chemical and materials sciences. Nature Synthesis, 2(6), 483-492.Link