How AI Drives Natural Product Drug Discovery
For centuries, nature has been our pharmacy, offering a wealth of compounds used in antibiotics, cancer therapies, and more. But as the demand for new drugs intensifies, so does the need to uncover new natural compounds hidden in the depths of the biological world. This is where artificial intelligence (AI) enters the scene, bringing advanced computational power to uncover and understand nature's chemical diversity like never before.
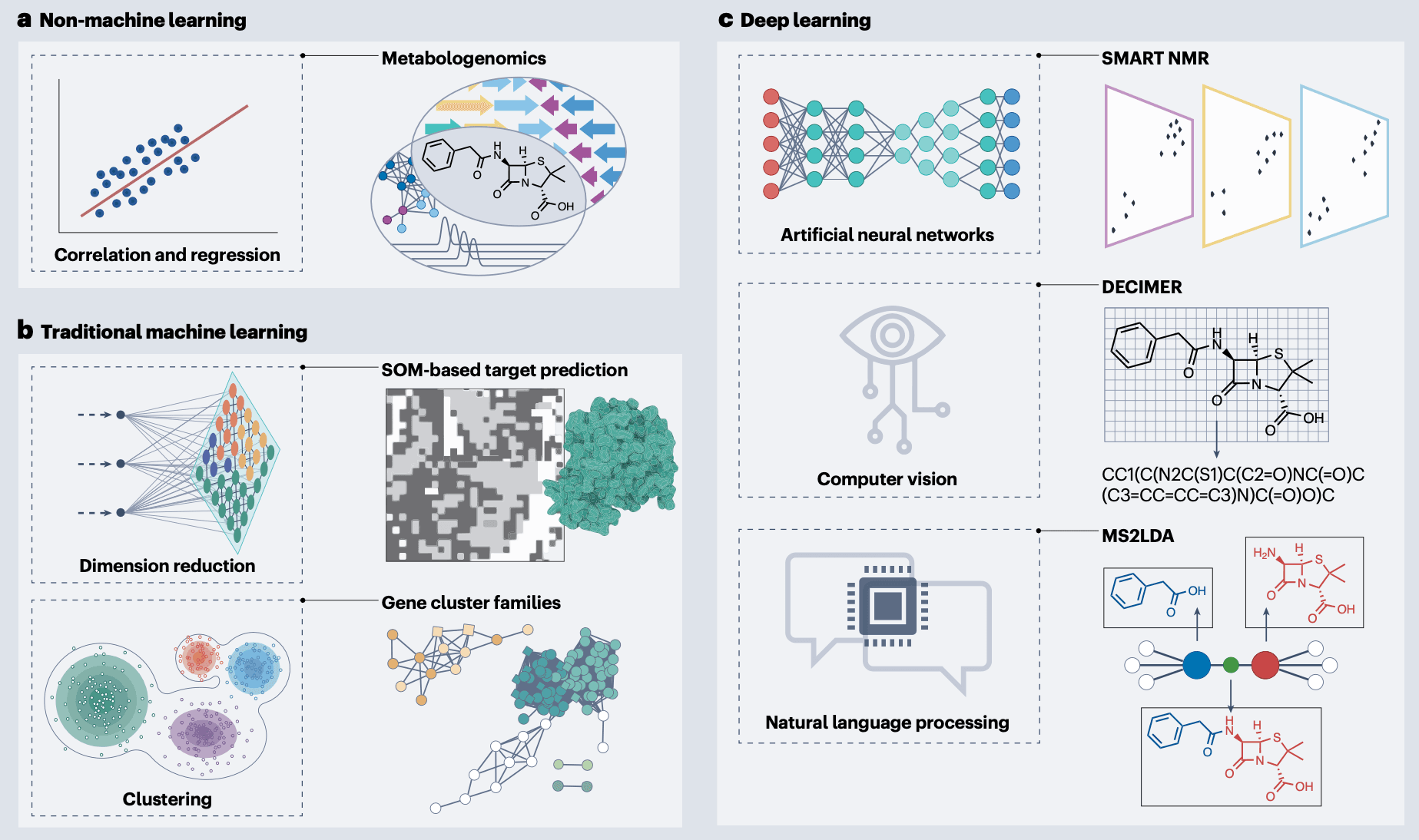
How Does AI Help Discover New Drugs from Natural Sources?
Imagine trying to find a needle in a haystack—that's what traditional natural product discovery can feel like. But AI turns that haystack into a map, showing where each needle might be hiding. Take a look at the figure above, which shows different types of AI applications in natural product drug discovery. These range from basic statistical methods to complex deep learning models [1].
On the left, we see non-machine learning methods like correlation and regression. These methods are used to link metabolomic data (essentially, a map of all the chemicals inside an organism) to genomic data (the genetic blueprint). While simple, these techniques help researchers spot relationships between genes and potential drug compounds in organisms.
How Do Traditional Machine Learning Techniques Aid in Discovery?
Moving a step further, traditional machine learning methods make natural product discovery even more insightful. In the figure's middle section, we see tools like self-organizing maps (SOMs) for target prediction, dimension reduction, and clustering of gene cluster families. This is where machine learning helps by organizing vast amounts of data, making it easier for scientists to spot patterns.
For example, clustering groups together similar gene families, which might share the ability to produce beneficial compounds. Dimension reduction is like shrinking down massive datasets to the core elements, making analysis faster and more efficient. These techniques help scientists identify clusters of genes that could be worth studying for their drug-producing potential, cutting down on time and resources needed for lab testing.
What Role Does Deep Learning Play in This Process?
The real magic happens in the deep learning section of the figure. Deep learning, the powerhouse of AI, can handle complex tasks such as predicting molecular structures, recognizing chemical images, and even interpreting natural language. Look at the right side of the figure: there are examples of artificial neural networks for analyzing complex NMR data, computer vision for automated image recognition, and natural language processing (NLP) for text mining.
One exciting example here is DECIMER, a deep learning tool for chemical structure recognition. By feeding it with thousands of chemical images, scientists can train DECIMER to interpret and convert these images into usable chemical information. This step is crucial because understanding a compound's structure gives clues about how it might work as a drug. Tools like DECIMER are already saving researchers countless hours that would have been spent manually analyzing chemical data.
Can AI Really Predict How These Compounds Will Act in the Body?
Yes! One of AI's most exciting roles in drug discovery is predicting the biological activity of natural products. AI models help researchers determine how a compound will interact with proteins and other cellular targets, a process that traditionally required extensive laboratory testing. By training algorithms on databases of known bioactivities and chemical structures, scientists can predict the pharmacological properties of new compounds, allowing them to focus on those with the highest therapeutic potential.
This application of AI extends to toxicity predictions, making it easier to avoid compounds with potential adverse effects early in the discovery process. AI can quickly assess the safety profile of a compound by analyzing patterns in toxicity data, saving time and resources.
What Challenges Lie Ahead for AI in Natural Product Drug Discovery?
Despite these advances, there are still significant challenges to address. One of the biggest is the need for high-quality, standardized data. AI algorithms depend on large, well-annotated datasets for training, but natural product data is often fragmented across various sources. To tackle this, initiatives are emerging to standardize data collection and integrate databases. Improved data quality will allow AI models to make more accurate predictions and continue advancing the field.
Another challenge is model validation. AI models can sometimes overfit data, leading to inaccurate predictions when applied to new compounds. Researchers are developing best practices to ensure that models are evaluated rigorously, making them more reliable in real-world applications.
What's Next for AI and Nature's Hidden Drug Compounds?
AI is rapidly advancing, and its integration with natural product research is just beginning. As computational methods evolve, researchers envision a future where AI can autonomously scan ecosystems for medicinal compounds, predict their structures, and assess their bioactivity, all within a virtual platform. This vision holds the potential to bring new, life-saving drugs to market faster and with more precision than ever before.
References
- Mullowney, M. W., et al. (2023). Artificial intelligence for natural product drug discovery. Nature Reviews Drug Discovery, 22(11), 895-916.Link