What Are Easy-to-Use AI Tools for Cancer Research?
A Guide for Non-Computational Cancer Research Scientists to Leverage the Power of Artificial Intelligence
The field of cancer research is undergoing a revolution, fueled by the rapid advancements in Artificial Intelligence (AI). While the term "AI" might conjure images of complex algorithms and extensive coding, the reality is that a new generation of user-friendly AI tools is emerging, making the power of AI accessible to every cancer researcher, regardless of their computational expertise.
The Challenge: Bridging the Gap Between Biology and Computation
Traditionally, cancer research and computational analysis have often existed in separate silos. Many cancer researchers, particularly those with a strong background in biology, may lack extensive programming experience, which can make it challenging to apply many new computer techniques. This digital divide can hinder the adoption of powerful AI-based approaches that could otherwise accelerate their research. Fortunately, the development of easy-to-use AI tools is bridging this gap, empowering non-computational biologists to leverage the capabilities of AI in their daily workflows.
Solution: User-Friendly AI Tools for Every Researcher
A growing number of AI-powered software platforms are specifically designed to be accessible to researchers without a strong computational background. These tools offer intuitive interfaces, often with drag-and-drop functionality and visual programming environments, eliminating the need to write complex code. Let's explore some of the most impactful tools:
1. For Image Analysis: QuPath and ilastik
Analyzing pathology images is a crucial aspect of cancer research. Two user-friendly tools have revolutionized this area:
QuPath
This open-source software is a powerhouse for whole-slide image analysis, providing tools for cell detection, tissue classification, and biomarker quantification. It has a point-and-click interface, making it easy to learn and use, even for those who have not previously done so [1].
Example: A researcher studying tumor heterogeneity could use QuPath to automatically segment different regions within a tumor sample, such as cancerous versus non-cancerous cells, and then quantify the expression of a specific biomarker in each region.
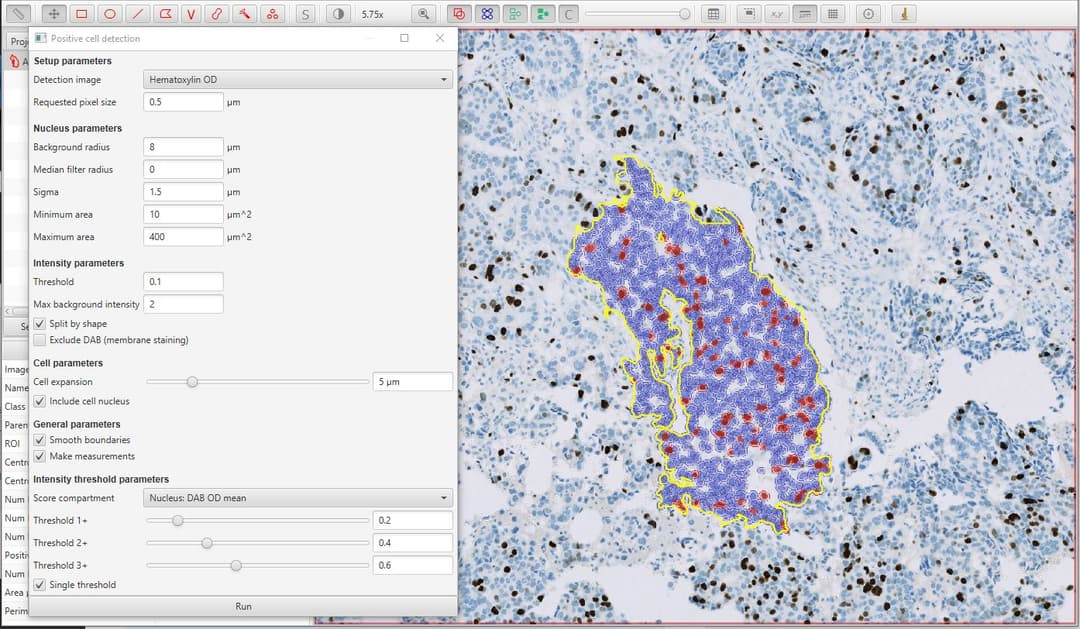
ilastik
This software specializes in interactive machine learning for image analysis. Users can train the software by manually annotating a small portion of their images, and ilastik then automatically applies these learned patterns to the rest of the dataset [2].
Example: A researcher could use ilastik to segment and quantify the number of blood vessels within a tumor sample by manually labeling a few examples of blood vessels and training ilastik to identify them throughout the image.
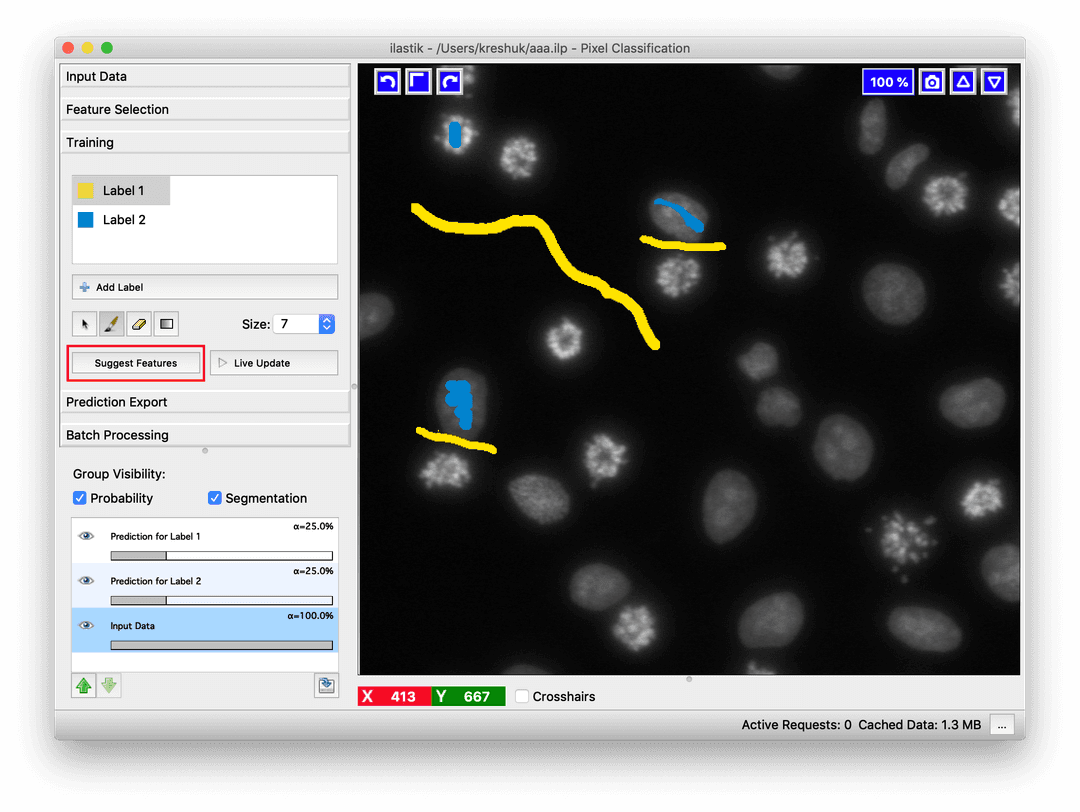
2. For Text Analysis: Natural Language Processing (NLP) Made Easy
The vast amount of information locked within research papers and clinical reports can be overwhelming. Natural Language Processing (NLP) which involves training a type of AI known as a Large Language Model (LLM) is transforming how we interact with this data. Here's how:
- LLMs like ChatGPT: While not specifically designed for research, LLMs are a new, AI-based technology that can be used to analyze scientific text and can quickly summarize papers, extract key information, and even help you write different sections of a paper by providing it with instructions in plain English.
- Specialized NLP tools: Platforms like Google Cloud's Healthcare Natural Language API offer pre-trained models specifically for extracting information from medical text.
3. For Genomics and Beyond: AI Platforms on the Rise
AI's impact extends beyond images and text, and is predicted to soon influence most types of cancer research. Platforms that simplify the application of AI to genomic data analysis and other complex datasets are becoming more readily available. For example:
- Galaxy: This open-source platform provides a web-based interface for a wide range of bioinformatics tools, many of which are now incorporating AI.
Addressing Potential Concerns: Limitations of AI
While the benefits of AI are clear, it is very important to be aware of potential limitations.
- "Black Box" Nature: Some AI models, especially deep learning models, can be difficult to interpret, and may appear as though they are operating like a "black box" where data goes in, and answers come out, but the internal decision-making process is not always fully understood. Using explainable AI (XAI) methods can help shed light on the decision-making process, but many methods remain "black boxes" for now.
- Data Bias: AI models are trained on data, and if that data reflects existing biases, the model's output may also be biased. For example, the authors of this review highlight that, as is true in this example, AI has traditionally been most heavily used in developed countries. The use of AI tools may therefore under-represent the global population in developing nations, or under-represent certain groups even within developed countries. Careful consideration of training data is crucial.
- Over-Reliance: AI should be seen as a tool to augment, not replace, human expertise. Critical evaluation of AI-generated results is always necessary.
- Data Sharing: Access to appropriate types of datasets that are compatible with AI methods is also important. One way this is accomplished is by standardizing the way that medical images are generated.
- Ethical Considerations: When using these tools to analyze patient data, it is important to prioritize patient privacy and adhere to all data-sharing regulations.
Getting Started: Your Journey into AI-Powered Research
Ready to explore the world of easy-to-use AI tools? Here are some steps to begin:
- Identify Your Needs: What are the specific tasks in your research that could benefit from automation or AI-powered analysis?
- Explore the Tools: Check out the websites of the tools mentioned above (QuPath, ilastik, ChatGPT, Google Cloud Healthcare Natural Language API, Galaxy).
- Start with Tutorials: Many of these tools offer excellent tutorials and documentation to get you started.
- Join Online Communities: Connect with other researchers using these tools to share knowledge and troubleshoot challenges.
The rise of user-friendly AI tools is democratizing access to powerful analytical capabilities, empowering cancer researchers of all computational backgrounds to accelerate their work and make new discoveries. The tools highlighted in this blog post represent just a glimpse of the exciting possibilities that AI offers. As the field continues to evolve, we can expect even more intuitive and powerful AI tools to emerge, further transforming the landscape of cancer research and ultimately improving patient outcomes. These advancements, as emphasized by Perez-Lopez et al. (2024), are not just about making research faster; they are about making it smarter, more insightful, and more impactful [3]. The future of cancer research is bright, and AI is playing a key role in illuminating the path forward.
References
- Bankhead, P. et al. QuPath: Open source software for digital pathology image analysis. Sci. Rep. 7, 16878 (2017).Link
- Berg, S., Kutra, D., Kroeger, T. et al. ilastik: interactive machine learning for (bio)image analysis. Nat Methods 16, 1226–1232 (2019).Link
- Perez-Lopez, R., Ghaffari Laleh, N., Mahmood, F., & Kather, J. N. (2024). A guide to artificial intelligence for cancer researchers. Nature Reviews Cancer.Link