Are We Over-Relying on AI in Science?
As AI revolutionizes scientific research, it's crucial to understand its limitations. This article delves into the hidden vulnerabilities of AI in science, exploring data insufficiency, planning limitations, and the illusion of understanding. Discover why human expertise remains irreplaceable in the scientific process.
What Makes AI Vulnerable in Scientific Research?
Artificial Intelligence is often seen as a game-changer for science, capable of processing data and generating insights at scales unimaginable for humans. But here's the catch: AI systems, especially those driven by large language models (LLMs), come with significant vulnerabilities that can compromise research quality [1].
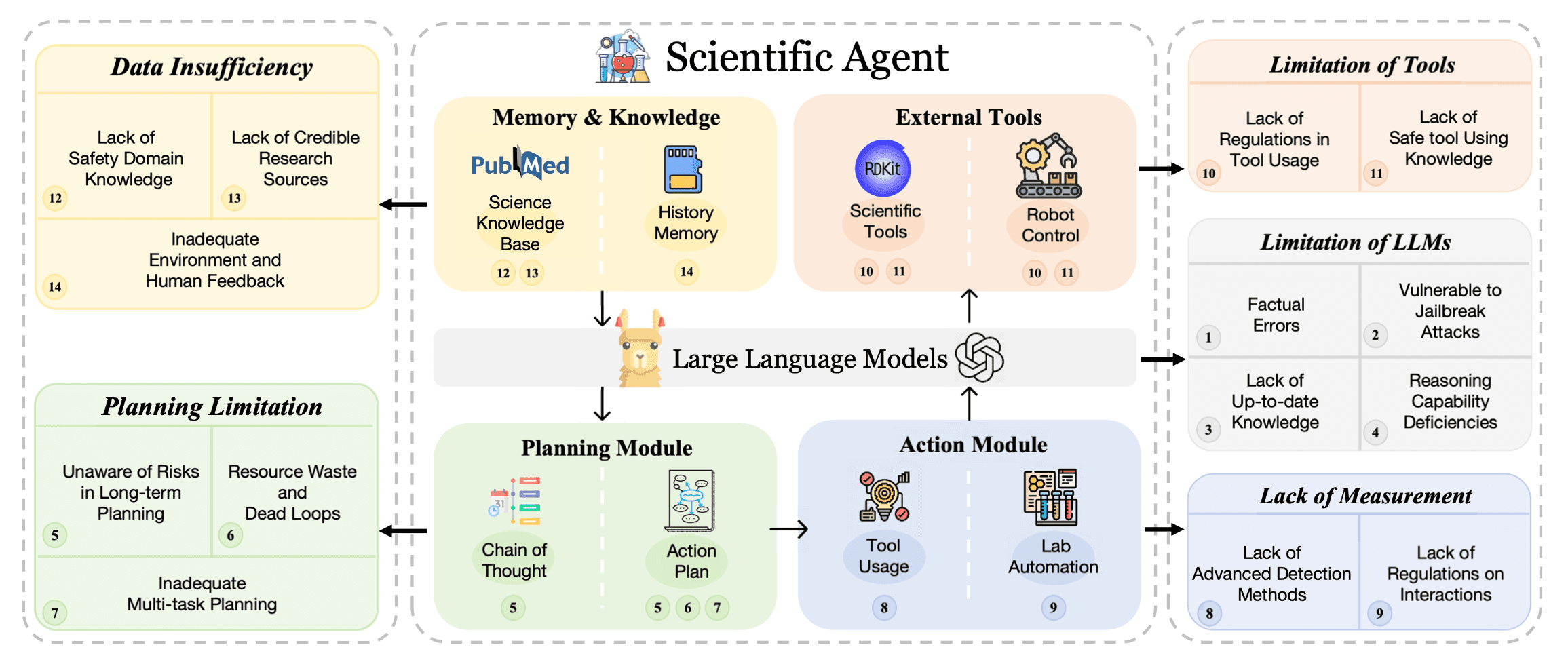
A recent study maps out the challenges faced by AI agents in autonomous scientific pipelines. These challenges span data insufficiency, planning limitations, tool constraints, the limitations of the LLMs themselves, and measurement gaps. For instance, many AI models lack access to up-to-date or credible scientific databases, and they often operate without necessary human feedback.
How Do AI-Driven Scientific Agents Fall Short?
Imagine AI as a scientific apprentice. It can quickly analyze data and follow instructions but lacks foresight and nuanced judgment. Scientific agents powered by AI often struggle with what we might call "planning limitations." While AI can help draft a plan of action, it often fails to consider long-term risks or unintended consequences, like resource waste or falling into repetitive cycles (dead loops).
Take, for example, multi-step experiments. If an AI agent cannot anticipate the implications of a particular action in the context of a broader project, it may end up consuming more resources than necessary or getting caught in endless testing loops without producing valuable insights. This is particularly problematic in complex scientific research where multiple factors are interdependent. In this sense, AI may be doing exactly what it's programmed to do, but without a broader understanding, it risks undermining the entire scientific endeavor.
Is AI Truly Capable of 'Understanding' Science?
One of the most compelling critiques of AI in research is its lack of genuine understanding—a concept explored in depth in a recently published Nature paper [2]. The authors introduce the notion of "illusions of understanding." This occurs when AI produces results that appear coherent and credible, but are fundamentally hollow because they lack a true grasp of the underlying scientific principles.
Just because an AI can generate plausible explanations or predictions does not mean it truly comprehends the science. This superficial understanding can lead to dangerous misconceptions and flawed research outcomes if not properly managed and overseen by human experts.
Why Does This Illusion of Understanding Matter?
The illusions of understanding created by AI are more than just an academic curiosity—they have real-world consequences. When scientists and researchers rely on AI-driven insights without verifying them through human expertise, they risk basing decisions on potentially flawed data or misinterpreted results. This can lead to erroneous conclusions in critical fields like healthcare, environmental science, and engineering, where the stakes are particularly high.
For instance, in medical research, an AI might suggest a treatment pathway based on pattern recognition rather than a nuanced understanding of patient context or disease complexity. Without human oversight, these recommendations could lead to ineffective or even harmful outcomes. The illusion of understanding can foster overconfidence in AI tools, encouraging a hands-off approach that's risky at best and dangerous at worst.
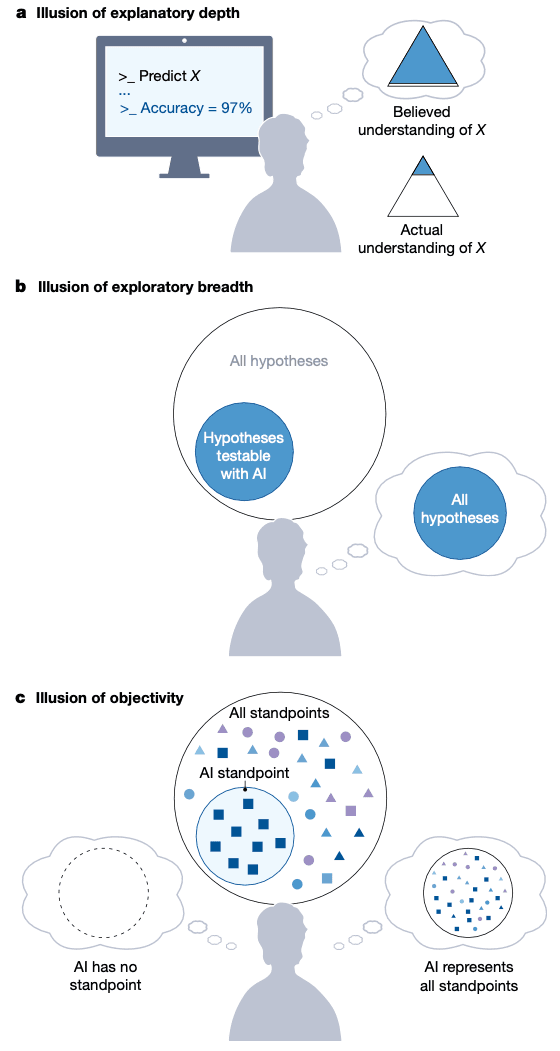
What Can We Do to Safeguard Science in the Age of AI?
As AI continues to make inroads into science, we must develop strategies to mitigate its limitations and safeguard research integrity. The first step is recognizing that AI, no matter how advanced, is not a substitute for human expertise. It is a powerful tool that can augment human capabilities, but it requires strict regulatory frameworks, robust feedback mechanisms, and ongoing human oversight.
We also need to invest in better training for AI models, ensuring they have access to comprehensive and current data sets. Regularly updated knowledge bases and adaptive learning algorithms can help improve AI's accuracy, but they won't eliminate the need for human judgment. Additionally, scientific organizations should prioritize transparency, making it clear when AI-driven insights are being used and highlighting the limitations of these insights.
In short, while AI has the potential to accelerate scientific discovery, we must tread carefully. We should not let the illusion of understanding lure us into complacency. By acknowledging AI's vulnerabilities and reinforcing the importance of human oversight, we can harness its strengths while minimizing the risks.